For this post I’m using the legacy cardinality estimator on SQL Server 2016 SP1.
The Problem
Scalar user defined functions are evil but sometimes necessary. The following scenario will sound a bit contrived but it’s based on a real world problem. Suppose that end users can filter the amount of data returned by a query by inputting values into a UDF that does some kind of translation. Below is a sample schema:
CREATE TABLE dbo.Example (
ID BIGINT NOT NULL,
NOT_ID VARCHAR(100) NOT NULL,
PRIMARY KEY (ID));
INSERT INTO dbo.Example WITH (TABLOCK)
(ID, NOT_ID)
SELECT TOP (1000000)
ROW_NUMBER() OVER (ORDER BY (SELECT NULL))
, REPLICATE('Example', 14)
FROM master..spt_values t1
CROSS JOIN master..spt_values t2;
GO
CREATE FUNCTION dbo.MY_FAVORITE_UDF (@ID BIGINT)
RETURNS BIGINT AS
BEGIN
RETURN @ID;
END;
Consider the following part of a much bigger query:
SELECT ID, NOT_ID
FROM dbo.Example
WHERE ID >= dbo.MY_FAVORITE_UDF(100000)
AND ID <= dbo.MY_FAVORITE_UDF(900000);
For this demo it’s not important that the UDF do anything so I must made it return the input. To keep things simple I’m not going to follow best practices around writing the query to avoid executing the UDFs for each row in the table. With the legacy cardinality estimator we get a cardinality estimate of 30% of the rows in the base table for each unknown equality condition. This means that a BETWEEN against two UDFs will give a cardinality estimate of 9%. The important point is that the cardinality estimate will not change as the inputs for the UDFs change, except for the trivial case in which the inputs are the same. This can easily be seen by varying the inputs and looking at the estimated execution plans:
SELECT ID, NOT_ID
FROM dbo.Example
WHERE ID >= dbo.MY_FAVORITE_UDF(100000)
AND ID <= dbo.MY_FAVORITE_UDF(900000);
Query plan:
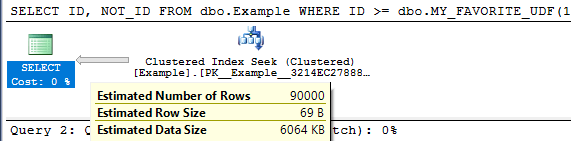
SELECT ID, NOT_ID
FROM dbo.Example
WHERE ID >= dbo.MY_FAVORITE_UDF(500000)
AND ID <= dbo.MY_FAVORITE_UDF(499999);
Query plan:
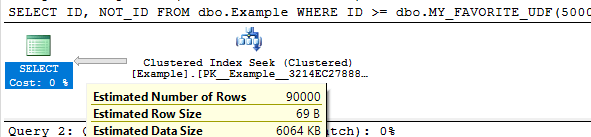
SELECT ID, NOT_ID
FROM dbo.Example
WHERE ID BETWEEN dbo.MY_FAVORITE_UDF(1)
AND dbo.MY_FAVORITE_UDF(1);
Query plan:
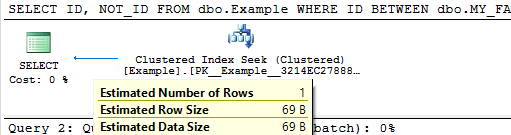
The cardinality estimate (CE) of just that simple query doesn’t really matter. But it could matter very much if that query was part of a larger query with other joins. The 9% estimate may not serve us well depending on the rest of the query and what end users tend to input. We might know that the end users tend to pick large or small ranges. Even if we don’t know anything about the end users, certain queries may do better with larger or smaller cardinality estimates.
Decreasing the Cardinality Estimate
Let’s suppose that we do some testing and find that a cardinality estimate of lower than 9% is the best choice for typical end user inputs. There are a few techniques available to decrease the cardinality estimate by a fixed percentage.
Method 1
First option is to use TOP PERCENT along with an OPTIMIZE FOR hint. I’m not really a fan of TOP PERCENT. The implementation always spools unless it gets optimized out with TOP (100) percent. It would be nice if it didn’t spool. Anyway, perhaps getting a different cardinality estimate is worth the spool. Below is one method to get a cardinality estimate of 3% of the base table:
DECLARE @top_percent FLOAT = 100;
SELECT TOP (@top_percent) PERCENT ID, NOT_ID
FROM dbo.Example
WHERE ID >= dbo.MY_FAVORITE_UDF(100000)
AND ID <= dbo.MY_FAVORITE_UDF(900000)
OPTION (OPTIMIZE FOR (@top_percent = 33.33333333));
Query plan:

The percent value is a float so we can go almost anywhere between 0 – 9% for the final estimate. However, if we have to use scalar UDFs in this fashion there’s a chance that we’re doing it to write platform agnostic code. The TOP trick here isn’t likely to work in other platforms.
Method 2
Suppose we add another inequality against a UDF that’s guaranteed not to change the results. 0.3^3 = 0.027 so we would expect an estimate of 2.7%. That is indeed what happens:
SELECT ID, NOT_ID
FROM dbo.Example
WHERE ID >= dbo.MY_FAVORITE_UDF(100000)
AND ID <= dbo.MY_FAVORITE_UDF(900000) -- redundant filter to change CE
AND ID > dbo.MY_FAVORITE_UDF(100000) - 1;
Query plan:
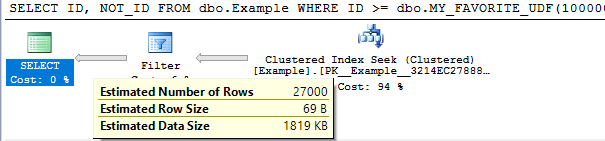
We can also mix things up with OR logic to make more adjustments. The query below has a fixed CE of 4.59%:
SELECT ID, NOT_ID
FROM dbo.Example
WHERE ID >= dbo.MY_FAVORITE_UDF(100000)
AND ID <= dbo.MY_FAVORITE_UDF(900000) -- redundant filter to change CE
AND (ID > dbo.MY_FAVORITE_UDF(100000) - 1
OR ID > dbo.MY_FAVORITE_UDF(100000) - 2);
Query plan:
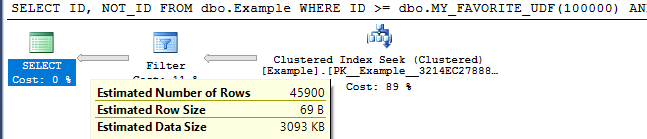
It should be possible to mix and match to get something close to the CE that you want. I need to reiterate that as the code is written this will lead to additional UDF executions per row. You can also use techniques with fixed CE that don’t involve UDFs if you’re confident that Microsoft won’t change the guesses for them (which for the legacy cardinality estimator is probably a pretty safe assumption at this point).
Increasing the Cardinality Estimate
In some cases we will want a cardinality estimate above 9%.
Method 1
The TOP PERCENT trick won’t work here since TOP on its own can’t increase a cardinality estimate. We can use OR logic with UDFs to raise the estimate. Consider this filter condition:
ID >= dbo.MY_FAVORITE_UDF(100000)
OR ID >= dbo.MY_FAVORITE_UDF(900000) - 1
The first inequality gives an estimate of 30% and the second inequality gives an estimate of (100% – 30%) * 30% = 21%. In total we would get an estimate of 51%. If we apply that twice we should get an overall estimate of 0.51 * 0.51 = 26.01% . This is indeed what happens:
SELECT ID, NOT_ID
FROM dbo.Example
WHERE (ID >= dbo.MY_FAVORITE_UDF(1)
OR ID >= dbo.MY_FAVORITE_UDF(1) - 1)
AND (ID <= dbo.MY_FAVORITE_UDF(2)
OR ID <= dbo.MY_FAVORITE_UDF(2) + 1);
Query plan:
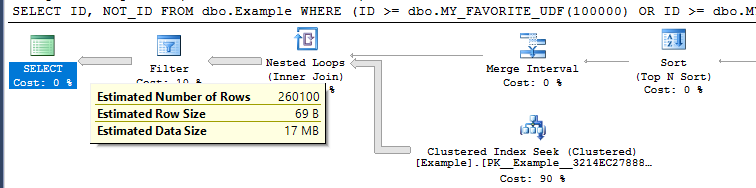
By adding more UDFs to the OR clauses we can increase the cardinality estimate further.
Method 2
For another way to do it we can take advantage of the fact that an inequality filter against a UDF has the same cardinality as the negated condition. That means that this:
SELECT ID, NOT_ID
FROM dbo.Example
EXCEPT
SELECT ID, NOT_ID
FROM dbo.Example
WHERE -- negate original expression
ID < dbo.MY_FAVORITE_UDF(100000) OR ID > dbo.MY_FAVORITE_UDF(900000);
Will return the same results as the original query but have a much higher cardinality estimate. Writing it in a better way, we see a cardinality estimate of ~54.4%:
SELECT e1.ID, e1.NOT_ID
FROM dbo.Example e1
WHERE NOT EXISTS (
SELECT 1
FROM dbo.Example e2
WHERE e1.ID = e2.ID
-- negate original expression
AND e2.ID < dbo.MY_FAVORITE_UDF(100000) OR e2.ID > dbo.MY_FAVORITE_UDF(900000)
);
Query plan:
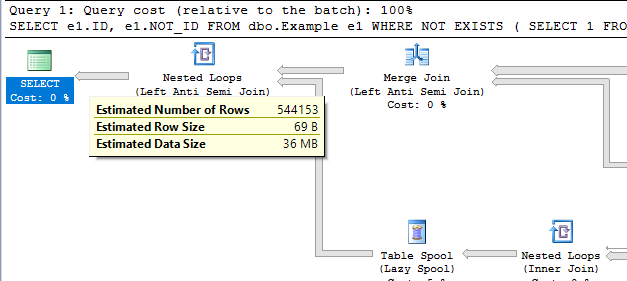
This can be adjusted up and down by adding additional UDFs. It comes with the cost of an additional join so it’s hard to think of an advantage of doing it this way.
Method 3
For a third option we can use the MANY() table-valued function developed by Adam Machanic. This function can be used to increase the cardinality estimate of a point in a plan by a whole number. If we want a cardinality estimate of 18% from the UDF it’s as easy as the following:
SELECT TOP (9223372036854775807) ID, NOT_ID
FROM dbo.Example
CROSS JOIN dbo.Many(2)
WHERE ID BETWEEN dbo.MY_FAVORITE_UDF(100000)
AND dbo.MY_FAVORITE_UDF(900000);
Query plan:
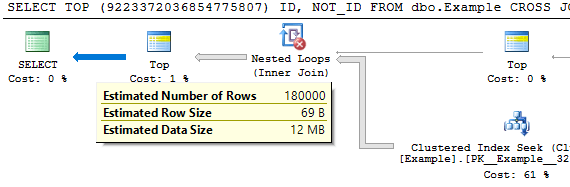
I added the superfluous TOP to prevent the MANY() reference from getting moved around in the plan. This method has the disadvantage that it may not be platform-agnostic.
Hopefully you never find yourself in a situation where you need to use tricks like this. Thanks for reading!
Going Further
If this is the kind of SQL Server stuff you love learning about, you’ll love my training. I’m offering a 75% discount to my blog readers if you click from here. I’m also available for consulting if you just don’t have time for that and need to solve performance problems quickly.