String aggregation is a not uncommon problem in SQL Server. By string aggregation I mean grouping related rows together and concatenating a string column for that group in a certain order into a single column. How will do CCIs do with this type of query?
The Data Set
A simple table with three columns is sufficient for testing. ITEM_ID is the id for the rows that should be aggregated together. LINE_ID stores the concatenation order within the group. COMMENT is the string column to be concatenated. The table will have 104857600 rows total with 16 rows per ITEM_ID.
CREATE TABLE dbo.CCI_FOR_STRING_AGG (
ITEM_ID BIGINT NOT NULL,
LINE_ID BIGINT NOT NULL,
COMMENT VARCHAR(10) NULL,
INDEX CCI CLUSTERED COLUMNSTORE
);
DECLARE @loop INT = 0
SET NOCOUNT ON;
WHILE @loop < 100
BEGIN
INSERT INTO dbo.CCI_FOR_STRING_AGG WITH (TABLOCK)
SELECT
t.RN / 16
, 1 + t.RN % 16
, CHAR(65 + t.RN % 16)
FROM
(
SELECT TOP (1048576)
(1048576 * @loop) - 1 +
ROW_NUMBER() OVER (ORDER BY (SELECT NULL)) RN
FROM master..spt_values t1
CROSS JOIN master..spt_values t2
) t
OPTION (MAXDOP 1);
SET @loop = @loop + 1;
END;
On my machine this codes takes around a minute and a half and the final table size is around 225 MB. I’m inserting batches of 1048576 rows with MAXDOP 1 to get nice, clean rowgroups.
The Test Query
Let’s concatenate the strings at an ITEM_ID level for all ITEM_IDs with an id between 3276800 and 3342335. All of the data is stored in a single rowgroup and the CCI was built in such a way that all other rowgroups can be skipped with that filter. This should represent the best case for CCI performance. A common way to concatenate a column is with the FOR XML PATH method:
SELECT
o.ITEM_ID
, STUFF(
(
SELECT ','+ i.COMMENT
FROM dbo.CCI_FOR_STRING_AGG i
WHERE o.ITEM_ID = i.ITEM_ID
ORDER BY i.LINE_ID
FOR XML PATH('')
)
,1 ,1, '') ALL_COMMENTS
FROM dbo.CCI_FOR_STRING_AGG o
WHERE o.ITEM_ID BETWEEN 3276800 AND 3342335
GROUP BY o.ITEM_ID;
Note that I’m not bothering with the additional arguments for the XML part, but you should whenever using this method in production.
The query plan looks reasonable at first glance:

However, the query takes nearly six minutes to complete. That’s not the lightning fast CCI query performance that we were promised!
Spool Problems
We can see from the actual execution plan that SQL Server scanned all 104.8 million rows from the CCI on a single thread:
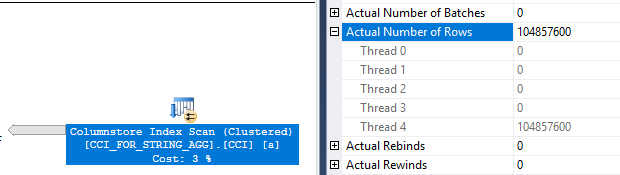
Those rows were then sent into an index spool which was read from all four threads in the nested loop. The CCI scan and the build of the index spool took the most time in the plan so it makes sense why CPU time is less than elapsed time, even with a MAXDOP 4 query:
CPU time = 305126 ms, elapsed time = 359176 ms.
Thinking back to how parallel nested loop joins work, it seems unavoidable that the index spool was built with just one thread. The rows from the outer result set are sent to the inner query one row at a time on different threads. All of the threads run independently at MAXDOP 1. The eager spool for the index is a blocking operation and the blocking occurs even when the subquery isn’t needed. Consider the following query in which the ITEM_ID = 3400000 filter means that rows from the FOR XML PATH part of the APPLY will never be needed:
SELECT o.ITEM_ID
, ac.ALL_COMMENTS
FROM
(
SELECT TOP (9223372036854775807) b.ITEM_ID
FROM dbo.CCI_FOR_STRING_AGG b
WHERE b.ITEM_ID BETWEEN 3276800 AND 3342335
GROUP BY b.ITEM_ID
) o
OUTER APPLY (
SELECT 'TEST'
UNION ALL
SELECT STUFF(
(
SELECT ','+ i.COMMENT
FROM dbo.CCI_FOR_STRING_AGG i
WHERE o.ITEM_ID = i.ITEM_ID
ORDER BY i.LINE_ID
FOR XML PATH('')
)
,1 ,1, '')
WHERE o.ITEM_ID = 3400000
) ac (ALL_COMMENTS)
OPTION (MAXDOP 4, QUERYTRACEON 8649);
The index spool is still built for this query on one thread even though the startup expression predicate condition is never met. It seems unlikely that we’ll be able to do anything about the fact that the index spool is built with one thread and that it blocks execution for the query. However, we know that we only need 1048576 rows from the CCI to return the query’s results. Right now the query takes 104.8 million rows and throws them into the spool. Can we reduce the number of rows put into the spool? The most obvious approach is to simply copy the filter on ITEM_ID into the subquery:
SELECT
o.ITEM_ID
, STUFF(
(
SELECT ','+ i.COMMENT
FROM dbo.CCI_FOR_STRING_AGG i
WHERE o.ITEM_ID = i.ITEM_ID
and i.ITEM_ID BETWEEN 3276800 AND 3342335
ORDER BY i.LINE_ID
FOR XML PATH('')
)
,1 ,1, '') ALL_COMMENTS
FROM dbo.CCI_FOR_STRING_AGG o
WHERE o.ITEM_ID BETWEEN 3276800 AND 3342335
GROUP BY o.ITEM_ID;
This doesn’t have the desired effect:
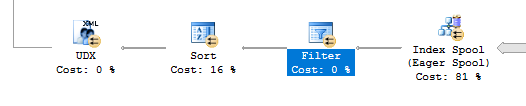
The filter is moved after the index build. We’ll still get all of the rows from the table put into the spool, but no rows will come out unless ITEM_ID is between 3276800 and 3342335. This is not helpful. We can get more strict with the query optimizer by adding a superfluous TOP to the subquery. That should force SQL Server to filter on ITEM_ID before sending rows to the spool because otherwise the TOP restriction may not be respected. One implementation:
SELECT
o.ITEM_ID
, STUFF(
(
SELECT ','+ i.COMMENT
FROM
(
SELECT TOP (9223372036854775807)
a.ITEM_ID, a.LINE_ID, a.COMMENT
FROM dbo.CCI_FOR_STRING_AGG a
WHERE a.ITEM_ID BETWEEN 3276800 AND 3342335
) i
WHERE o.ITEM_ID = i.ITEM_ID
ORDER BY i.LINE_ID
FOR XML PATH('')
)
,1 ,1, '') ALL_COMMENTS
FROM dbo.CCI_FOR_STRING_AGG o
WHERE o.ITEM_ID BETWEEN 3276800 AND 3342335
GROUP BY o.ITEM_ID;
SQL Server continues to outsmart us:

As you can see in the plan above, the spool has completely disappeared. I was not able to find a way, even with undocumented black magic, to reduce the number of rows going into the spool. Perhaps it is a fundamental restriction regarding index spools. In fact, we can use the undocumented trace flag 8615 to see that spools are not even considered at any part in the query plan for the new query. On the left is the previous query with the spool with an example highlighted. On the right is the new query. The text shown here is just for illustration purposes, but we can see the spool on the left:
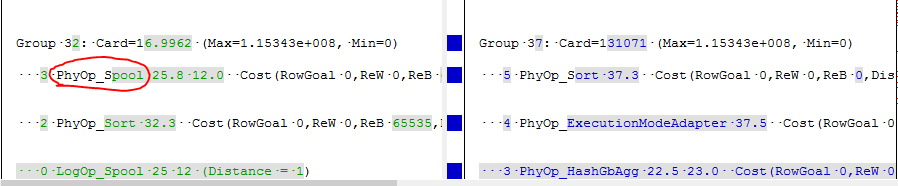
The important point is that for this query we appear to be stuck.
Rowgroup Elimination Problems
We can try our luck without the spool by relying on rowgroup elimation alone. The spool can’t be eliminated with the NO_PERFORMANCE_SPOOL hint, but another option (other than the TOP trick above) is to use the undocumented QUERYRULEOFF syntax to disable the optimizer rule for building spools:
SELECT
o.ITEM_ID
, STUFF(
(
SELECT ','+ i.COMMENT
FROM dbo.CCI_FOR_STRING_AGG i
WHERE o.ITEM_ID = i.ITEM_ID
ORDER BY i.LINE_ID
FOR XML PATH('')
)
,1 ,1, '') ALL_COMMENTS
FROM dbo.CCI_FOR_STRING_AGG o
WHERE o.ITEM_ID BETWEEN 3276800 AND 3342335
GROUP BY o.ITEM_ID
OPTION (QUERYRULEOFF BuildSpool);
The spool is gone but we don’t get rowgroup elimination:
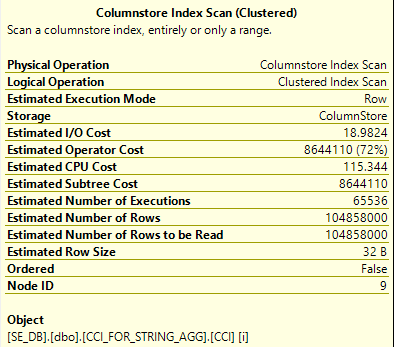
We can get rowgroup elimination even without hardcoded filters with a bitmap from a hash join. Why don’t we get it with a nested loop join? Surely SQL Server ought to be able to apply rowgroup elimination for each outer row as it’s processed by the inner part of the nested loop join. We can explore this question further with tests that don’t do string aggregation. The query below gets rowgroup elimination but the constant filters are passed down to the CCI predicate:
SELECT *
FROM (VALUES (1), (2), (3), (4)) AS v(x)
INNER JOIN dbo.CCI_FOR_STRING_AGG a ON v.x = a.ITEM_ID
OPTION (FORCE ORDER, LOOP JOIN);
If we throw the four values into a temp table:
SELECT x INTO #t
FROM (VALUES (1), (2), (3), (4)) AS v(x)
The join predicate is applied in the nested loop operator. We don’t get any rowgroup elimination. Perhaps TOP can help us:
SELECT *
FROM #t v
CROSS APPLY (
SELECT TOP (9223372036854775807) *
FROM dbo.CCI_FOR_STRING_AGG a
WHERE v.x = a.ITEM_ID
) a
OPTION (LOOP JOIN);
Sadly not:
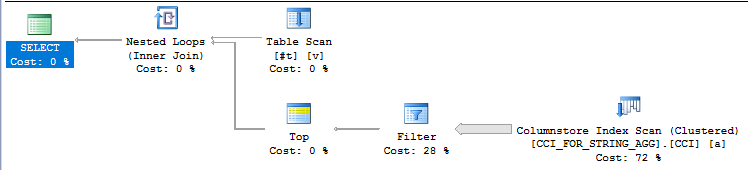
The join predicate is applied in the filter operation. We still don’t get any rowgroup elimination. Frustratingly, we get the behavior that we’re after if we replace the CCI with a heap:
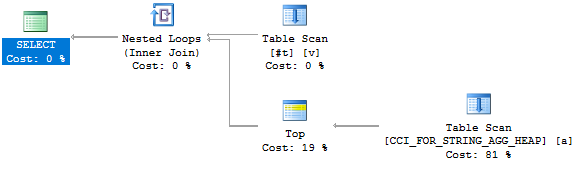
I don’t really see an advantage in pushing down the predicate with a heap. Perhaps Microsoft did not program the optimization that we’re looking for into the query optimizer. After all, this is likely to be a relatively uncommon case. This query is simple enough in that we filter directly against the CCI. In theory, we can give our query a fighting chance by adding a redundant filter on ITEM_ID to the subquery. Here’s the query that I’ll run:
SELECT
o.ITEM_ID
, STUFF(
(
SELECT ','+ i.COMMENT
FROM dbo.CCI_FOR_STRING_AGG i
WHERE o.ITEM_ID = i.ITEM_ID
and i.ITEM_ID BETWEEN 3276800 AND 3342335
ORDER BY i.LINE_ID
FOR XML PATH('')
)
,1 ,1, '') ALL_COMMENTS
FROM dbo.CCI_FOR_STRING_AGG o
WHERE o.ITEM_ID BETWEEN 3276800 AND 3342335
GROUP BY o.ITEM_ID
OPTION (QUERYRULEOFF BuildSpool);
Unfortunately performance is even worse than before. The query finished in around 51 minutes on my machine. It was a proper parallel query and we skipped quite a few rowgroups:
Table ‘CCI_FOR_STRING_AGG’. Segment reads 65537, segment skipped 6488163.
CPU time = 10028282 ms, elapsed time = 3083875 ms.
Skipping trillions of rows is impressive but we still read over 68 billion rows from the CCI. That’s not going to be fast. We can’t improve performance further with this method since there aren’t any nonclustered indexes on the CCI, so there’s nothing better to seek against in the inner part of the loop.
Temp Tables
We can use the humble temp table to avoid some of the problems with the index spool. We’re able to insert into it in parallel, build the index in parallel, and we can limit the temp table to only the 1048576 rows that are needed for the query. The following code finishes in less than two seconds on my machine:
CREATE TABLE #CCI_FOR_STRING_AGG_SPOOL (
ITEM_ID BIGINT NOT NULL,
LINE_ID BIGINT NOT NULL,
COMMENT VARCHAR(10) NULL
);
INSERT INTO #CCI_FOR_STRING_AGG_SPOOL WITH (TABLOCK)
SELECT a.ITEM_ID, a.LINE_ID, a.COMMENT
FROM dbo.CCI_FOR_STRING_AGG a
WHERE a.ITEM_ID BETWEEN 3276800 AND 3342335;
CREATE CLUSTERED INDEX CI_CCI_FOR_STRING_AGG_SPOOL
ON #CCI_FOR_STRING_AGG_SPOOL (ITEM_ID, LINE_ID);
SELECT
o.ITEM_ID
, STUFF(
(
SELECT ','+ i.COMMENT
FROM #CCI_FOR_STRING_AGG_SPOOL i
WHERE o.ITEM_ID = i.ITEM_ID
ORDER BY i.LINE_ID
FOR XML PATH('')
)
,1 ,1, '') ALL_COMMENTS
FROM dbo.CCI_FOR_STRING_AGG o
WHERE o.ITEM_ID BETWEEN 3276800 AND 3342335
GROUP BY o.ITEM_ID;
The query plan for the last query:
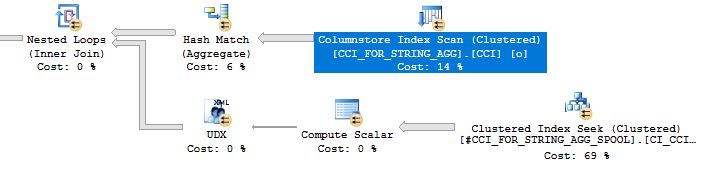
With this approach we’re not really getting much from defining the underlying table as a CCI. If the table had more columns that weren’t needed then we could skip those columns with column elimination.
Other Solutions
An obvious solution is simply to add a covering nonclustered index to the table. Of course, that comes with the admission that columnstore currently doesn’t have anything to offer with this type of query. Other solutions which are likely to be more columnstore friendly include using a CLR function to do the aggregation and the SQL Server 2017 STRING_AGG function.
Note that recursion is not likely to be a good solution here. Every recursive query that I’ve seen involves nested loops. The dreaded index spool returns:
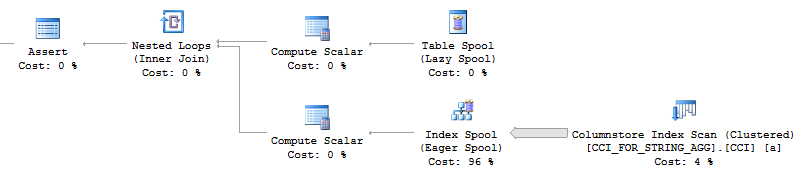
Final Thoughts
Note that the poor performance of these queries isn’t a problem specific to columnstore. Rowstore tables can have the similar performance issues with string aggregation if there isn’t a sufficient covering index. However, if CCIs are used as a replacement for all nonclustered indexes, then the performance of queries that require the table to be on the inner side of a nested loop join may significantly degrade. Some of the optimizations that make querying CCIs fast appear to be difficult to realize for these types of queries. The same string aggregation query when run against a table with a rowstore clustered index on ITEM_ID and LINE_ID finished in 1 second. That is an almost 360X improvement over the CCI.
Thanks for reading!
Going Further
If this is the kind of SQL Server stuff you love learning about, you’ll love my training. I’m offering a 75% discount to my blog readers if you click from here. I’m also available for consulting if you just don’t have time for that and need to solve performance problems quickly.